Sensor data from wearable devices analyzed over five years reveals walking and posture differences that predict fall risk in Parkinson’s patients.
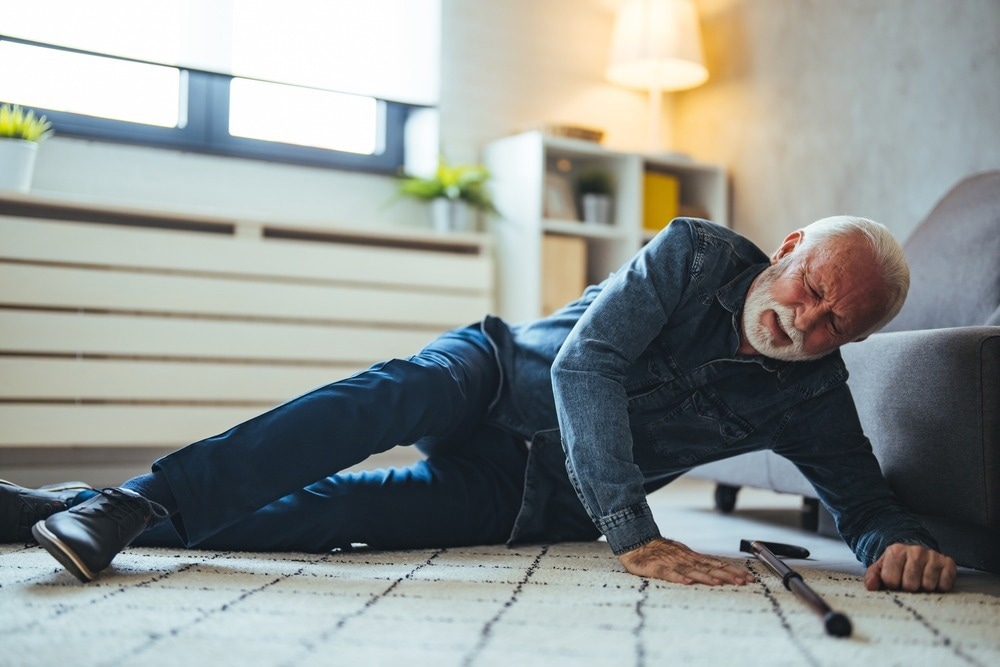
In a recent study published in Npj Digital Medicine, a research team from the University of Oxford explored how brief wearable sensor data assessments combined with machine learning models can predict fall risk in individuals with Parkinson’s disease for up to five years. By analyzing walking and postural sway, the research aimed to offer a reliable, objective method to anticipate falls and improve preventive care and clinical outcomes.
Background
Falls are a significant concern in Parkinson’s disease, often leading to injuries, reduced mobility, and diminished quality of life. Research shows that over half of individuals with Parkinson’s disease experience at least one fall, with increasing risks due to gait variability, postural instability, and disease progression.
Traditional fall risk evaluations rely heavily on clinical judgment, which can be subjective and inconsistent. However, emerging wearable sensor technologies provide an opportunity to measure movement more objectively, offering insights into gait and balance irregularities that are difficult to detect visually.
Previous studies have demonstrated the utility of wearable devices for short-term fall prediction, but most studies have focused on retrospective data on falls or have limited follow-up durations. Furthermore, the feasibility of short, clinic-based assessments to predict falls over extended periods remains unexplored, resulting in a lack of practical, scalable solutions for proactive management.
About the study
In the present study, the researchers examined 104 individuals with Parkinson’s disease as part of the longitudinal Oxford Quantification in Parkinsonism or OxQUIP cohort study. The participants were recruited based on specific criteria, including mild-to-moderate idiopathic Parkinson’s disease and the ability to walk and stand unassisted.
Baseline data were collected using wearable sensors during a two-minute walking task and a 30-second postural sway task. All participants wore six inertial measurement unit (IMU) sensors placed on their wrists, feet, sternum, and lumbar region to capture accelerometer, gyroscope, and magnetometer data.
The researchers determined fall status through clinical visits and follow-ups at two and five years. To ensure robust analysis, they resampled most of the “non-faller” class to balance the dataset for machine learning models. Five classifiers — Random Forest, Logistic Regression, ElasticNet, Support Vector Machine, and XGBoost — were trained using cross-validation techniques. Additional performance metrics included accuracy, precision, recall, and receiver operating characteristic curve-area under the curve (ROC-AUC) values.
The study also conducted feature selection to identify critical predictors, focusing on gait variability and postural sway. The impact of including clinicodemographic data such as age, disease duration, and baseline clinical scores was evaluated by testing four feature sets.
Furthermore, the researchers also assessed the predictive capability of kinematic features alone and in combined datasets using various models and ensured that all the analyses accounted for data standardization and avoided biases such as data leakage.
The goal of the study was to develop reliable, short-duration assessments for long-term fall prediction in Parkinson’s disease by integrating wearable technology with advanced statistical methods to enhance clinical decision-making.
Major findings
The findings reported that wearable sensors and machine learning models effectively predicted fall risk in individuals with Parkinson’s disease over time. At 24 months, the machine learning classifiers demonstrated excellent performance, with accuracy ranging between 84% and 92% and an area under the curve (AUC) exceeding 0.90.
For the five-year predictions, the Random Forest model, which incorporated clinicodemographic data, including age, achieved the highest accuracy of 78% with an AUC of 0.85. Furthermore, the researchers noted that adding clinicodemographic data marginally improved the predictive performance compared to kinematic features alone.
Gait and postural variability were identified as the most significant predictors of future falls. Additionally, major variables included the variability of single and double limb support phases, stride length, and postural sway acceleration. The study also found that shorter prediction horizons yielded higher model accuracy, furthermore highlighting the challenges of forecasting outcomes over extended periods due to variability in disease progression.
The performance of machine learning models at predicting falls was compared to clinical scales, such as the Movement Disorders Society (MDS) Modified Unified Parkinson’s Disease Rating Scale (MDS-UPDRS) and Parkinson’s Disease Questionnaire (PDQ-39).
The findings suggested that sensor-based assessments provide greater predictive accuracy. While some decline in prediction accuracy was observed for longer timeframes, the results demonstrated the potential of wearable technology to enhance fall risk management in clinical settings.
Conclusions
Overall, the study highlighted the potential of integrating wearable sensor data with machine learning models for predicting fall risk in Parkinson’s disease. The findings also emphasized the importance of walking and postural variability as predictive factors and demonstrated the feasibility of short-duration, clinic-based assessments.
By improving early detection of fall risks, these methods offer a pathway toward targeted interventions, reducing the incidence of falls and improving the quality of life for Parkinson’s disease patients.
- Sotirakis, C., Brzezicki, M. A., Patel, S., Conway, N., FitzGerald, J. J., & Antoniades, C. A. (2024). Predicting future fallers in Parkinson’s disease using kinematic data over a period of 5 years. Npj Digital Medicine, 7(1), 345. doi:10.1038/s41746024013115 https://www.nature.com/articles/s41746-024-01311-5